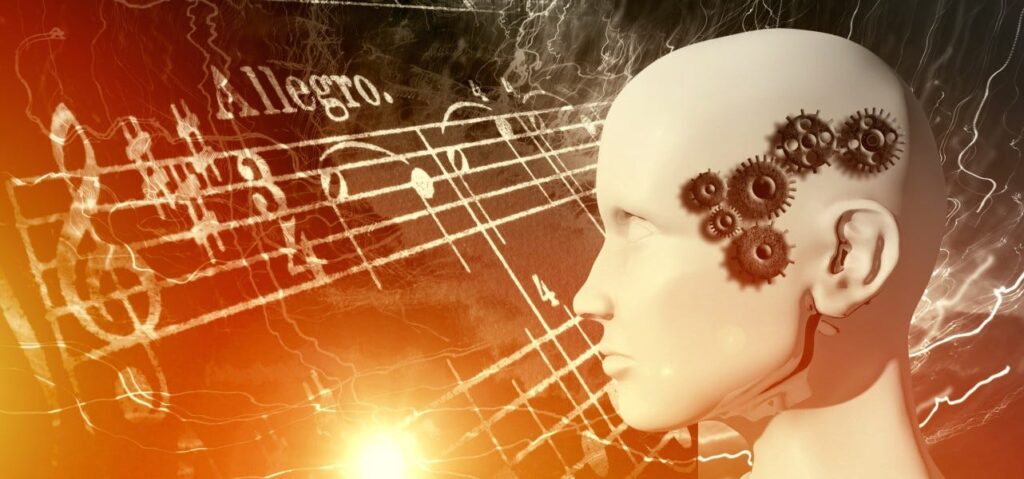
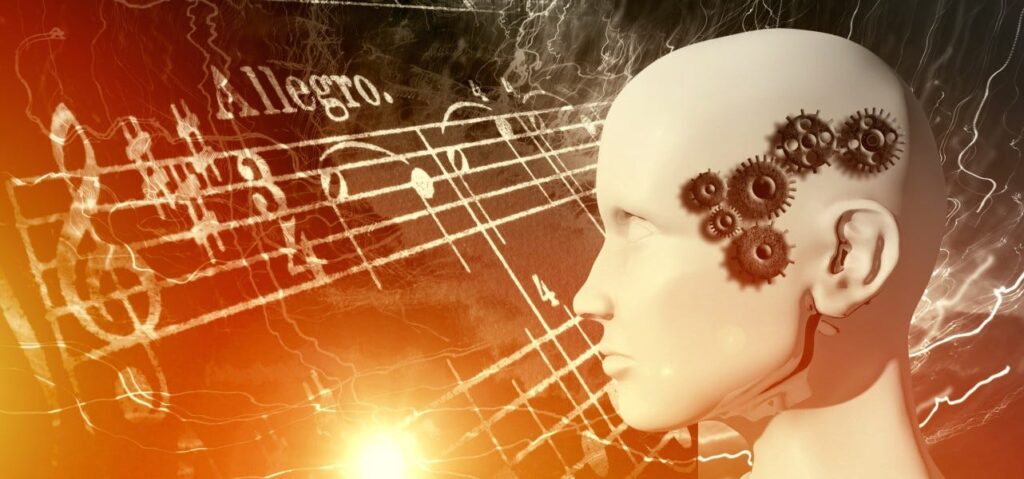
Analyzing musical compositions is essential for learning about their inner workings, patterns, and qualities. Recent years have seen remarkable progress in music analysis thanks to the introduction of machine learning methods.
The use of artificial intelligence (AI) in music production has potential. Machine learning algorithms, and in particular deep neural networks, are increasingly being used to examine massive music databases in order to inspire new compositions.
The first step in using AI to compose music is to train the machine learning (ML) algorithm on an existing music dataset, which might be a large collection of songs in a specific genre or style. The algorithm analyzes the chord progressions, melodies, beats, rhythms, and instrumentation of the song in order to generate new music with similar characteristics.
The article delves into the exciting area where music analysis and machine learning meet, illuminating how these tools are changing our perception of music and providing exciting new opportunities for musicians and music lovers alike.
Automatic Music Transcription


Automatic transcription of music is now possible because to machine learning algorithms, which take the useful information from audio recordings and turn it into musical notation. These algorithms can recognize notes, rhythms, chords, and other musical aspects by examining the audio input and using methods including signal processing, pattern recognition, and deep learning. In the fields of music education, musicology, and digital music libraries, automatic music transcription can be used to speed up processes like score generation, music search, and database analysis.
Music Genre Classification
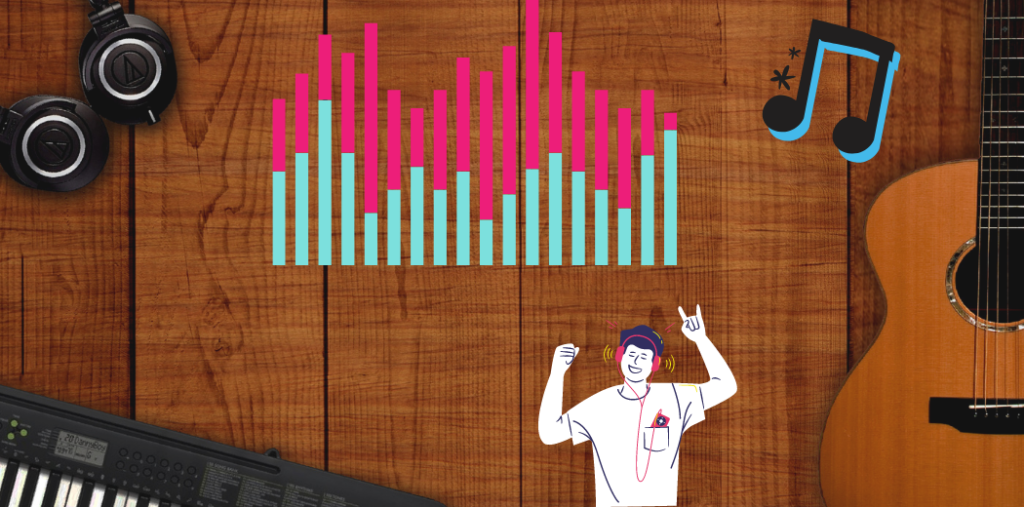
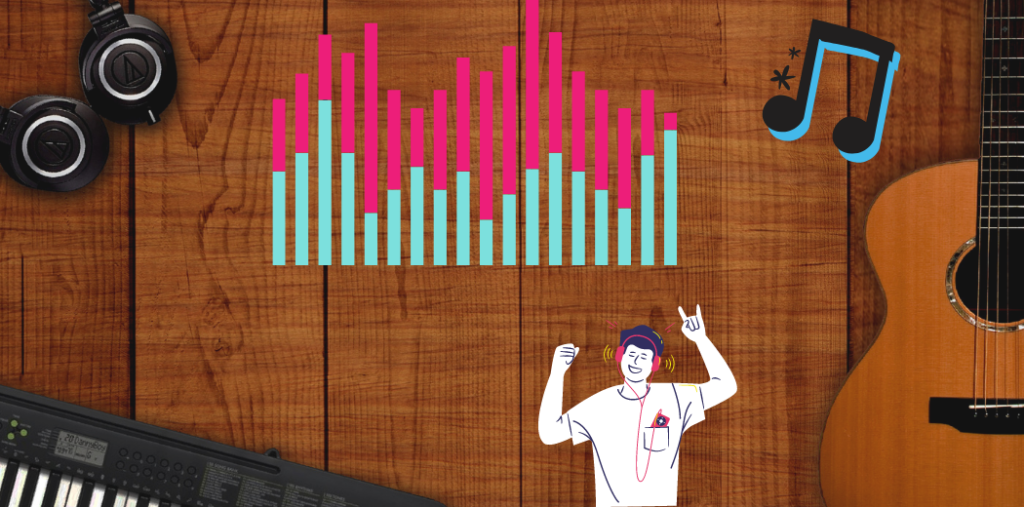
Songs can be automatically categorized by using machine learning algorithms to divide music into various genres based on audio data. These algorithms are capable of accurately identifying musical songs by training on labeled datasets and extracting properties including timbre, rhythm, and tonality. When it comes to music recommendation systems, streaming services, and the organizing of music information, genre classification is useful since it enables users to find new music that matches their tastes.
Music Emotion Recognition
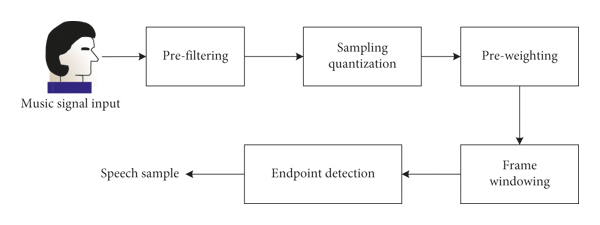
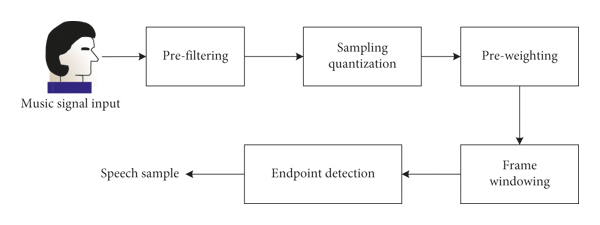
The emotional content of music can be identified and categorized using machine learning algorithms that examine auditory data. These algorithms may deduce emotions like happiness, sadness, enthusiasm, or tranquillity by extracting auditory properties like pace, pitch, and dynamics. In music therapy, where certain emotions are desired to be elicited or managed, music emotion detection has applications in mood-based music recommendations, personalized playlists, and music therapy.
Music Structural Analysis
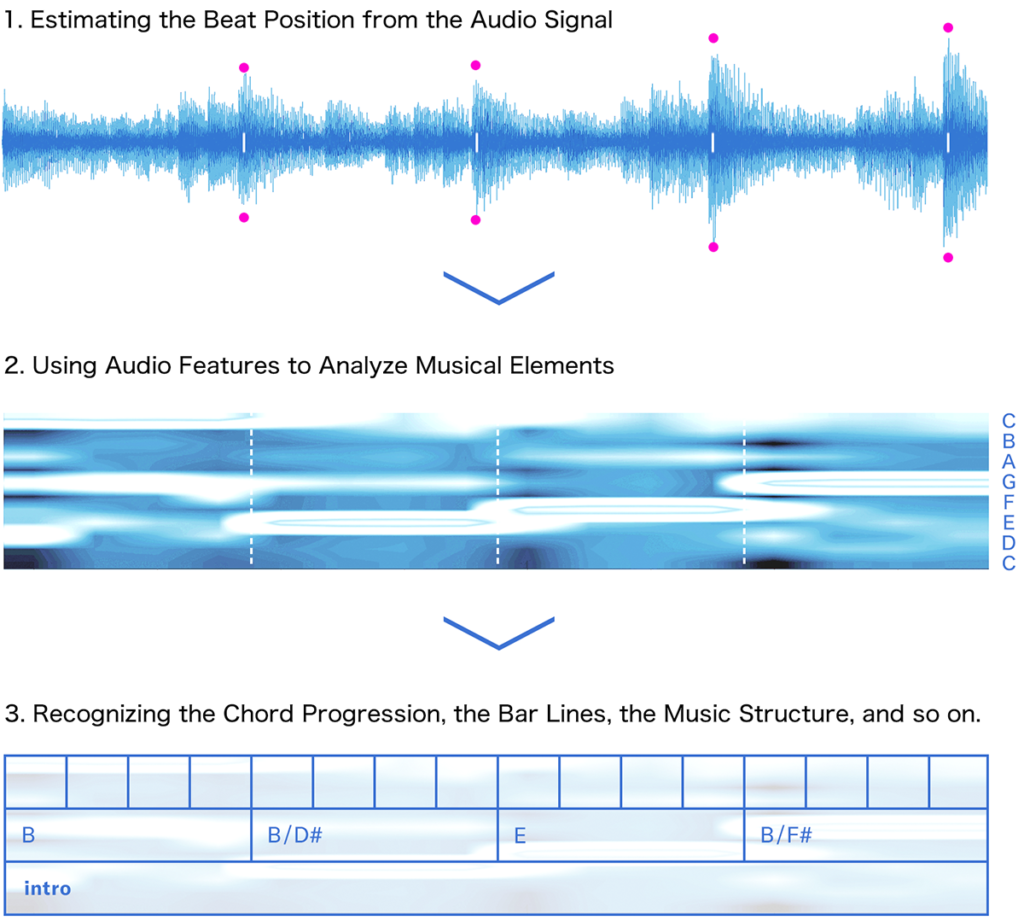
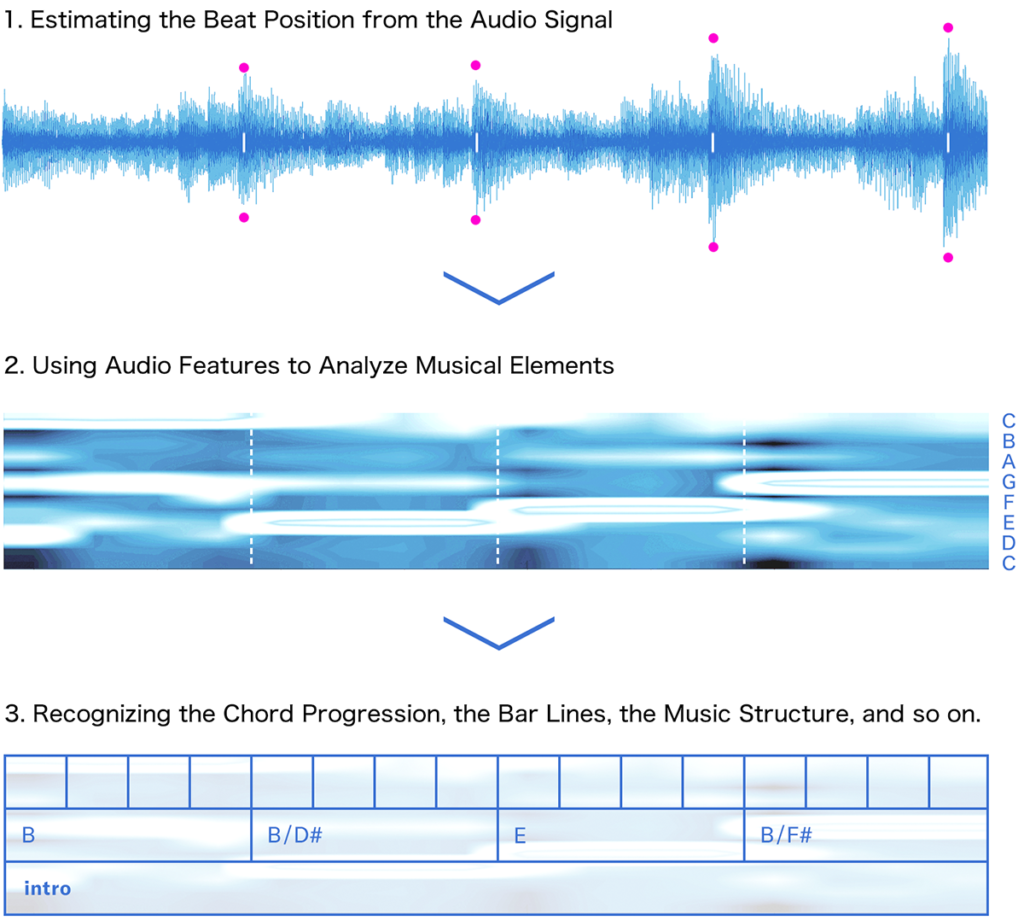
Analyzing the form and structure of music is made easier by machine learning algorithms. A musical composition can be divided into sections by algorithms, which can also spot recurrent patterns, identify key shifts, and recognize harmonic progressions. Machine learning models can help in music creation, arrangement, and knowledge of musical styles by examining the correlations between musical parts and offering insightful information about musical composition.
Music Generation and Style Transfer
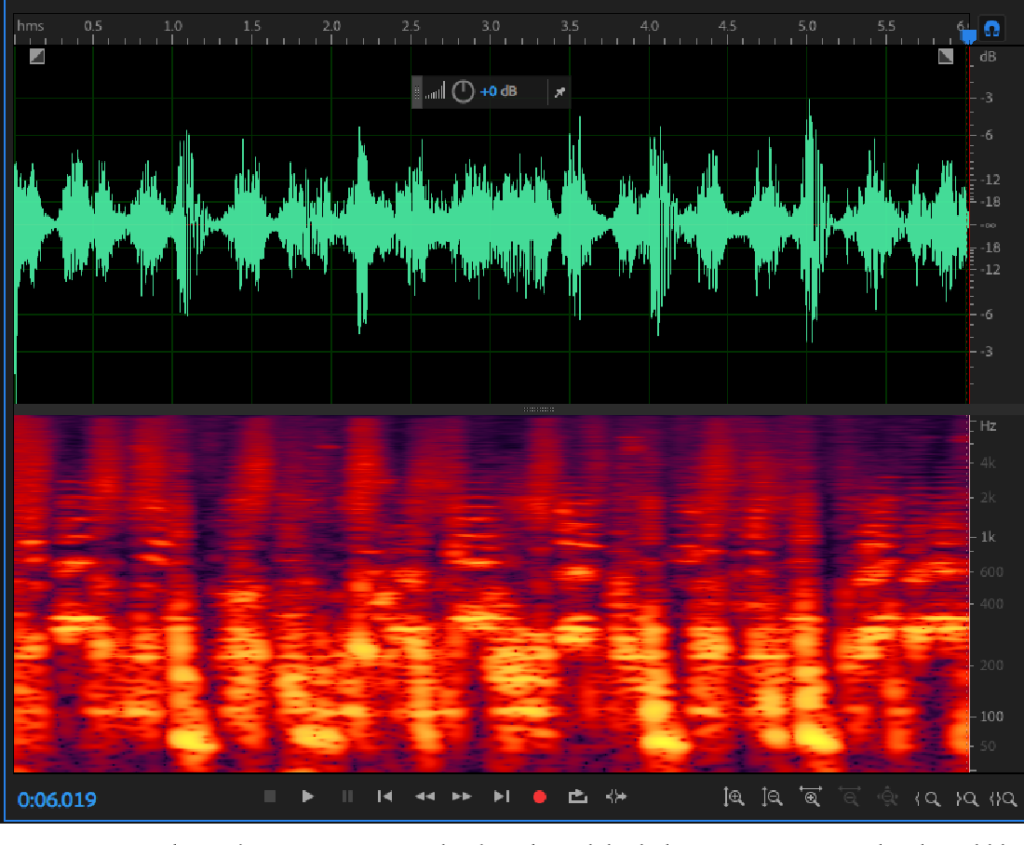
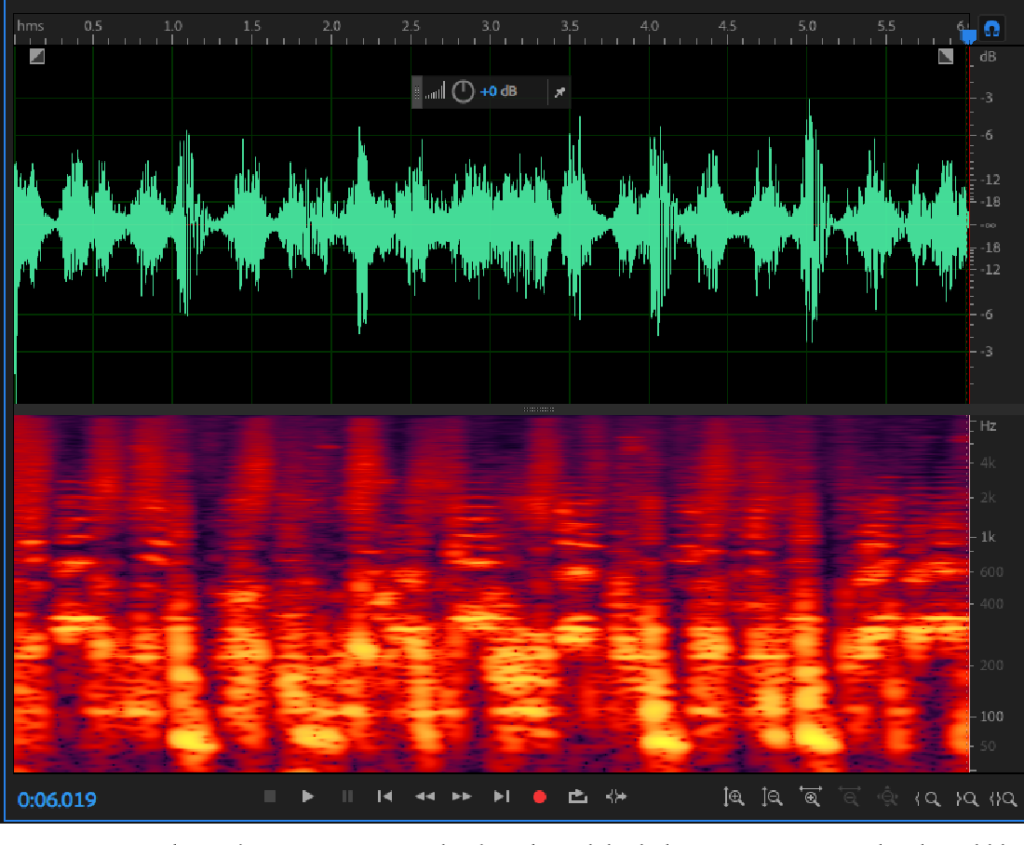
New musical compositions can be created using existing music datasets and machine learning models, especially generative models like Variational Autoencoders (VAEs) and Generative Adversarial Networks (GANs). These models can produce new music in the same vein as the style, melodic patterns, and harmonic structures of particular composers or genres. The construction of soundtracks, the generation of new music, and creative experimentation are all impacted by style transfer and music generation.
Music Recommendation Systems
Systems that recommend customized playlists and musical tracks based on listener preferences, listening history, and contextual data are powered by machine learning algorithms. In order to improve music discovery and user engagement, these systems analyze user behavior, music metadata, and collaborative filtering algorithms to generate individualized music suggestions.
Conclusion
The way we listen to, comprehend, and engage with music has changed as a result of the integration of music analysis and machine learning approaches. Machine learning has achieved important advancements in a number of fields, including automatic music transcription, genre classification, emotion recognition, structural analysis, music production, and recommendation systems. We may anticipate more developments in music analysis as technology advances, providing greater insights into the creation, performance, and listening habits of music. With machine learning as a strong ally, the music industry is set for ground-breaking innovations and fascinating possibilities.
Swikriti Dandotia